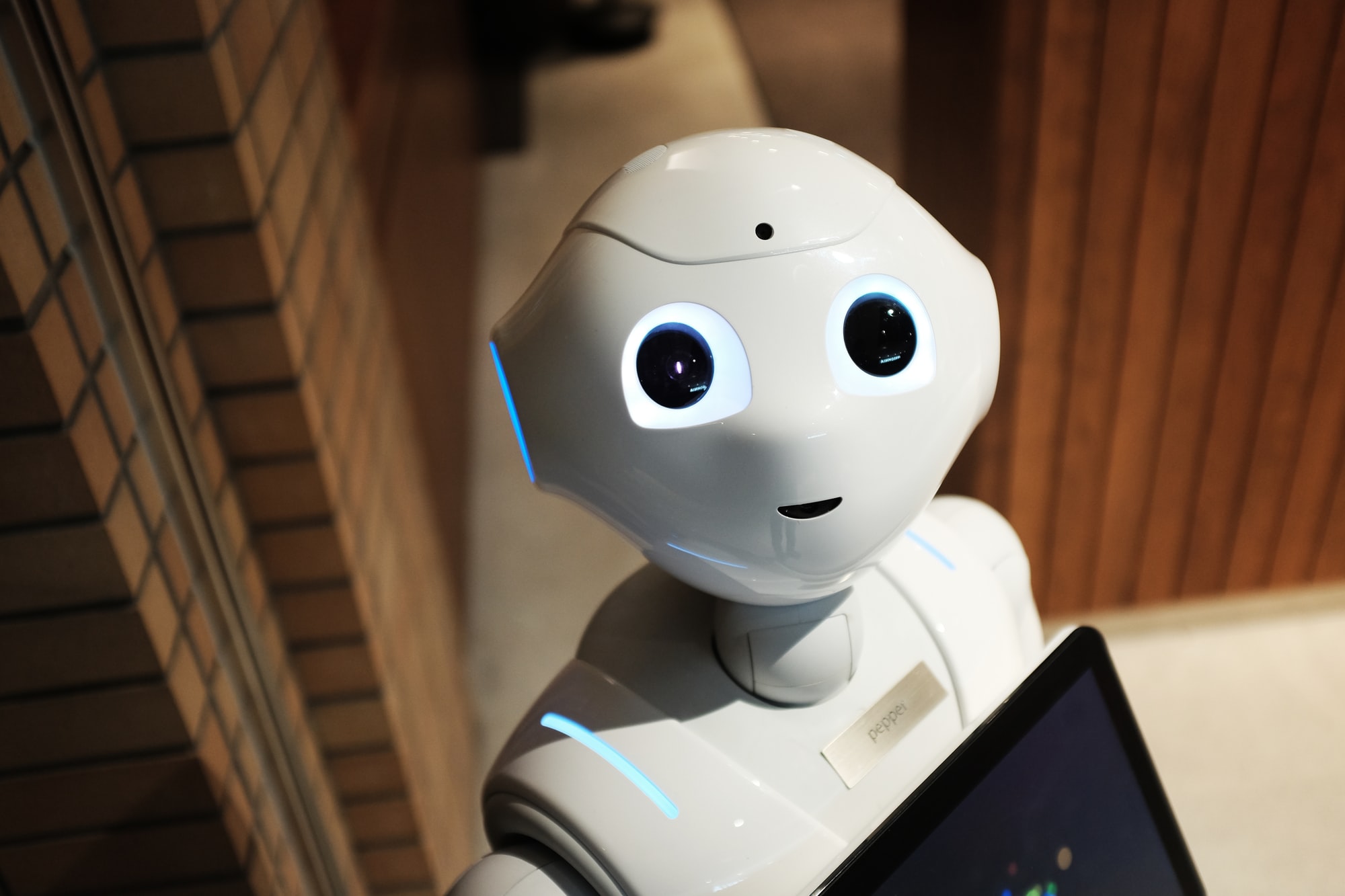
Artificial intelligence: sounds great, but where do we start?
AI and machine learning have long been the stuff of science fiction – but they are now becoming a reality in both the corporate and government arenas.
I have participated in my fair share of Discoveries and Inceptions in the past, both as a facilitator and as a contributor. Over the past year, though, there has been a new question asked in some of the Inceptions I’ve run – what is the potential for us to employ Artificial Intelligence (AI)? It is impossible to browse through a newspaper without stumbling upon an article expounding the benefits that AI and machine learning are now delivering.
Understandably, there is a substantial amount of scepticism about the immediate impacts of the new technology – much of the news is about prototypes that may not have a practical application, or may not deliver a meaningful return on investment for several years.
That said, AI is already part of the standard toolset of many trailblazing technology companies.
Running an Inception for an AI project is a bit different, and I would like to share my take on it. In particular, there are three things that immediately surface when exploring the potential for AI in new initiatives:
1. Greater ambiguity
Inceptions always start with uncertainty. We use the various workshop sessions to reduce the unknowns and get closer to a degree of certainty. In my experience, unless you are integrating with a well-established product, the level of the ambiguity around AI raises these unknowns to a new level.
Although some products, such as TensorFlow, Theano and Torch, have a degree of maturity, most solutions in the market are “hot off the press”. This will generally also be the case when you engage with AI startups to help build your solutions. You may end up being the first organisation to solve some of the “unknown unknowns” for your industry!
Be prepared to be comfortable working with lots of guesses and assumptions, and then course-correct continuously as you experiment and learn.
2. Training data
If you are integrating a deep-learning component into your product, you will need to train it first. Which immediately raises the question: “do we have enough in-house data for the training?”
For example, if your product is about distinguishing car makes from one another, you need to have enough car photos with various models and colours, taken from different angles. If your dataset does not exist, you might need to think about creating one (or just buy one).
After you create or acquire the data, you need to start thinking about how to store and access it. Depending on the problem and the need, training data can be massive.
Finally, you probably want to gather and store new data from your production systems as well, because nobody wants a product that cannot learn and improve over time. Your Architecture, Data and DevOps teams should collaborate to design the right data management solution for your organisation.
3. Rapid improvements in integration and solution development
If your product has elements of AI included, you are working with one of the fastest-changing technologies around. By the time you come to deploy your product, a better solution or technology may have emerged since you had your Inception.
Always be ready to accommodate these rapid shifts in the tools and technology. In your Inception, plan for frequent, small product releases that will provide the right amount of business agility to respond to the rapidly-changing underlying technology.
Our robot overloads may still be far away, but the reality is that AI and machine learning are already having a significant impact in a wide range of industries. They should both be in the consideration set when you plan your next Inception.
Next Paradigm has worked with numerous organisations to explore the potential for AI in their industry. For an initial discussion about how AI could benefit your organisation, contact us at info@nexxt.com.au.
Download